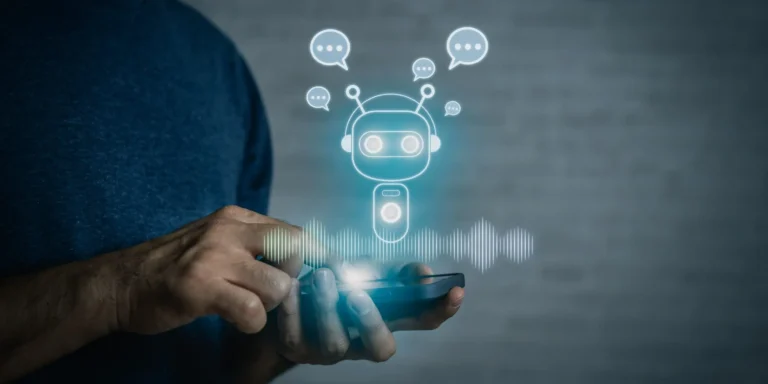
Understanding sentiment analysis allows businesses to make informed decisions grounded in measurable emotional data. By leveraging advanced NLP (natural language processing) and machine learning (ML) technologies, organisations can decode how their audience truly feels—whether it’s about products, services, or overall brand perception.
In industries like e-commerce, healthcare, and finance, where perception can significantly affect bottom lines, analysing sentiment isn’t optional—it’s essential. For example, a retail chain could use aspect-based sentiment analysis to identify negative reviews related to product delivery times, while a financial firm could track public sentiment shifts to inform trading strategies.
Convert Data Into Real-Time Insights
In an environment where over 500,000 tweets and 2 million Google searches occur every minute, actionable insights must come at speed. Real-time monitoring identifies mood shifts before they become critical, such as detecting a drop in brand sentiment within a customer complaint thread.
A practical streaming service could use sentiment analysis to evaluate social media reactions to a newly released show and make quick content adjustments, such as extending episodes or improving accessibility options, to address viewer concerns.
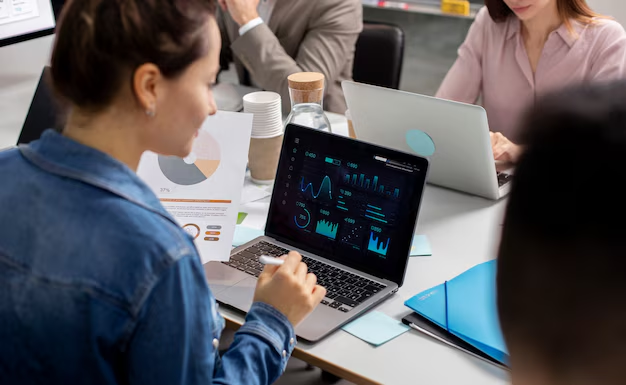
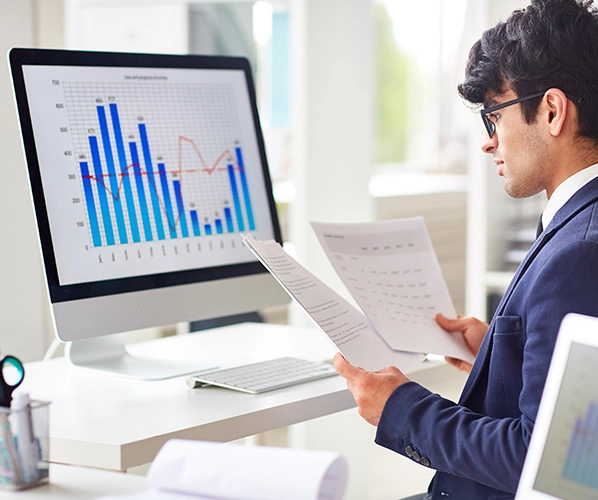
Gain Industry-Specific Insights
Pearl Lemon AI’s solutions dive deep into the unique requirements of your sector. For hospitality, this might involve analysing guest reviews to determine whether issues arise from staff behaviour, room cleanliness, or food quality. E-commerce companies might examine social media mentions to isolate product-specific complaints, enabling faster stock or manufacturing adjustments.
Keyword tagging, polarity detection, and intent classification ensure every piece of data serves a clear purpose—whether predicting churn or informing quarterly planning.
How Sentiment Analysis Works
The process begins with cleaning and organising data into manageable formats, followed by applying advanced algorithms to identify sentiments. Pearl Lemon AI applies hybrid sentiment analysis models, combining rule-based systems and deep learning for accuracy rates above 90%.
Our system does more than measure whether feedback is positive or negative. It identifies nuances, such as sarcasm or mixed emotions, and assigns measurable sentiment scores. These scores help decision-makers prioritise responses and allocate resources effectively.
Preprocessing for Accuracy
To extract clean, actionable data, our systems perform tokenisation, stop-word removal, and lemmatisation. This ensures irrelevant elements, such as filler words, are removed before analysis begins. Clean data reduces errors by as much as 35%, ensuring higher accuracy.
For instance, a sportswear brand might upload customer reviews for sentiment analysis. Our preprocessing eliminates repetitive terms like “um,” “maybe,” or “nice,” focusing on meaningful words like “sizing issue” or “defective zipper.”
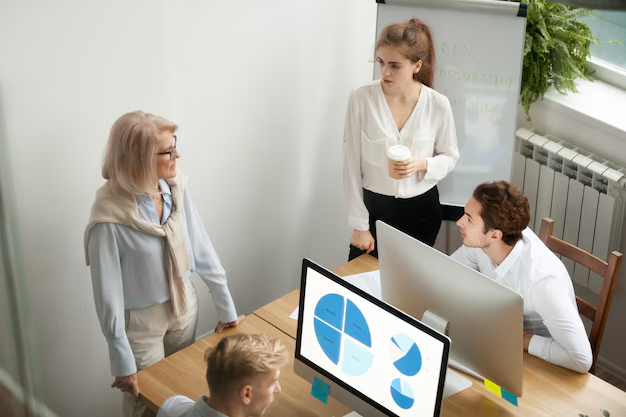
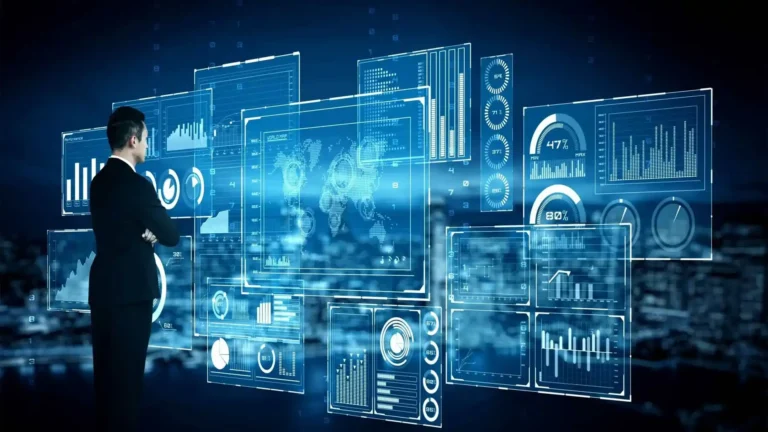
Real-Time Monitoring for Immediate Action
Our systems monitor live data streams from platforms such as Twitter, Reddit, or even internal chat logs, producing actionable insights within seconds. Businesses can react to public complaints, detect potential PR crises, or refine marketing campaigns without delays.
A telecommunications provider might use sentiment tracking during service outages, responding proactively to mitigate brand damage and update affected customers with resolutions.
Industries Transformed by Sentiment Analysis
Sentiment analysis has become a key driver for businesses looking to stay competitive. By extracting emotional and contextual data from feedback, companies can identify trends, resolve pain points, and improve customer experience. Industries like e-commerce, healthcare, and finance are reaping measurable benefits from leveraging these insights.
E-commerce: Convert Feedback Into Revenue
Retailers who actively use sentiment analysis to process customer reviews and social mentions experience up to 30% higher retention rates. Negative comments about slow delivery times, for example, can prompt operational adjustments that keep customers satisfied.
Case Study: An online marketplace might analyse the sentiment of product-specific reviews and discover that 20% of complaints mention inaccurate sizing. By addressing the issue at the manufacturer level, the retailer avoids future complaints and improves buyer trust.
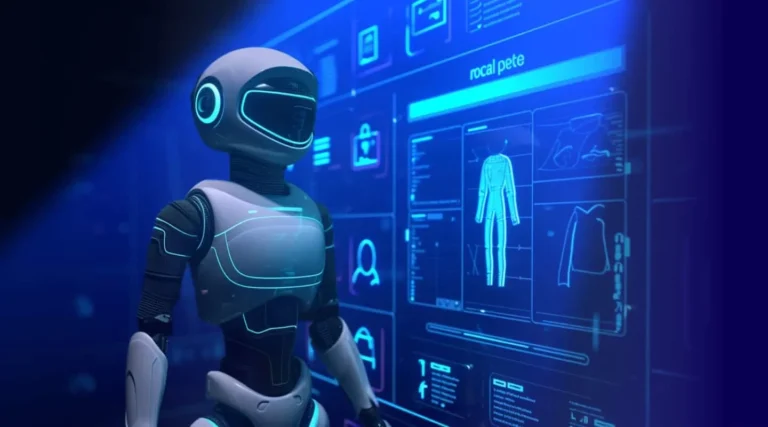
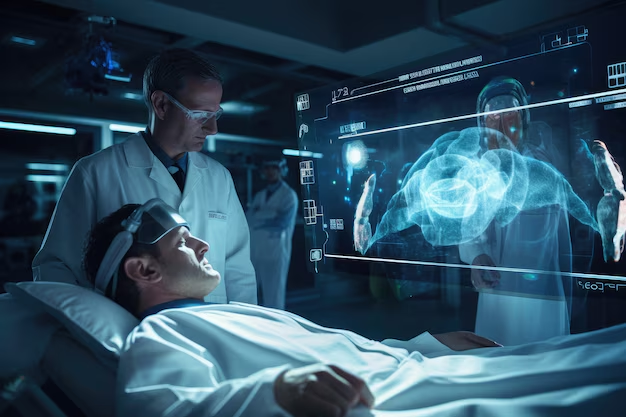
Healthcare: Understand Patient Sentiment
Healthcare providers use sentiment analysis to track emotional cues in patient feedback, improving services while identifying operational weaknesses. For example, a hospital might analyse patient surveys and find dissatisfaction around waiting times, prompting workflow changes that cut average wait durations by 15 minutes.
This approach is especially beneficial in tracking compliance metrics such as HCAHPS scores, enabling care providers to improve their performance in regulatory evaluations.
Financial Services: Manage Risks and Opportunities
Financial institutions rely on sentiment analysis to measure public opinion about market trends, products, or competitors. For instance, a firm tracking sentiment around emerging tech stocks might notice increased positivity, leading them to adjust portfolio recommendations.
A bank could monitor customer feedback to identify friction points in loan application processes, using this insight to design smoother user experiences.
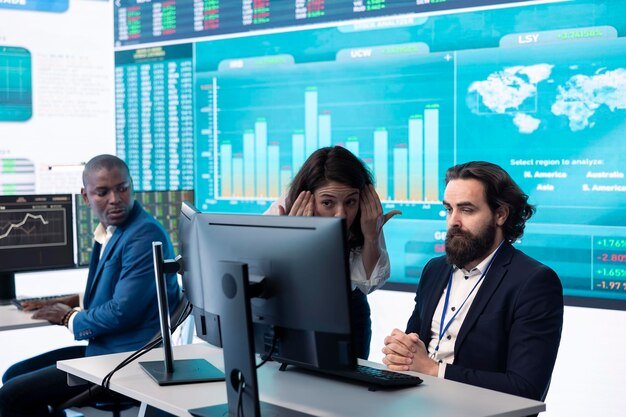
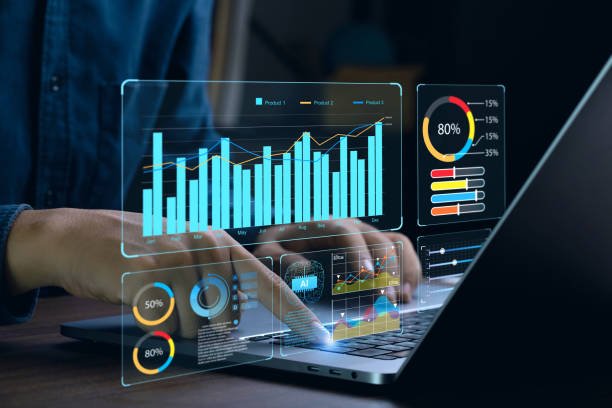
Overcoming Sentiment Analysis Challenges
Sentiment analysis isn’t without its obstacles. Complex language structures, cultural nuances, and the sheer variety of input formats can make interpretation challenging. Advanced techniques like deep learning, contextual embeddings, and rule-based systems help mitigate these challenges, ensuring precise and actionable insights.
Decoding Sarcasm and Ambiguity
Sarcasm and ambiguous statements can distort sentiment results if not handled properly. By integrating hybrid models—which combine rule-based logic with machine learning algorithms—we achieve higher accuracy in detecting sarcastic or ironic tones. For example, a sarcastic comment like “Amazing service, as always—still waiting for my package!” is flagged as negative, ensuring sentiment results reflect true customer intent.
We’ve achieved sarcasm detection accuracy rates above 90% in controlled datasets.
Handling Multilingual Datasets
Global businesses often face challenges with multi-language feedback. Our systems incorporate tools like XLM-RoBERTa to support over 20 languages while maintaining sentiment accuracy above 85%.
An international retailer tracking feedback in French, Japanese, and English can view unified sentiment results, ensuring no insights are lost across regions.
Managing Polarised Sentiments
Feedback containing both positive and negative points requires careful analysis. Using aspect-level sentiment analysis, we isolate sentiment tied to specific aspects. For example, in a review stating, “The food was great, but the service was slow,” we can separate sentiments for food and service.
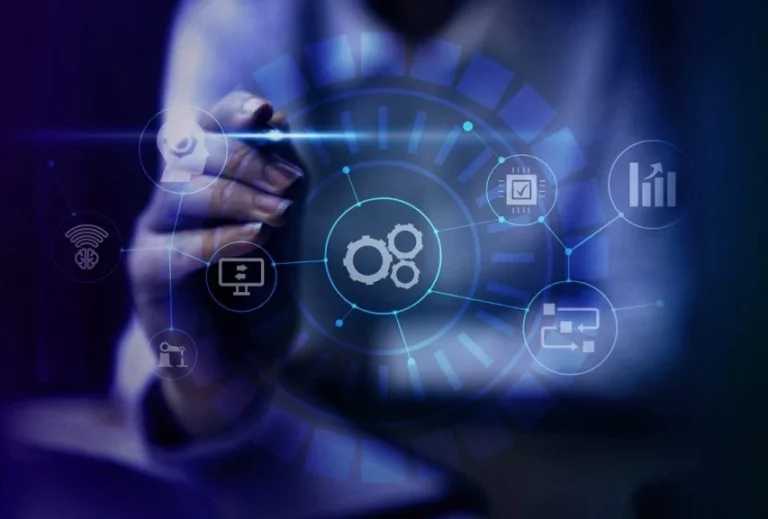
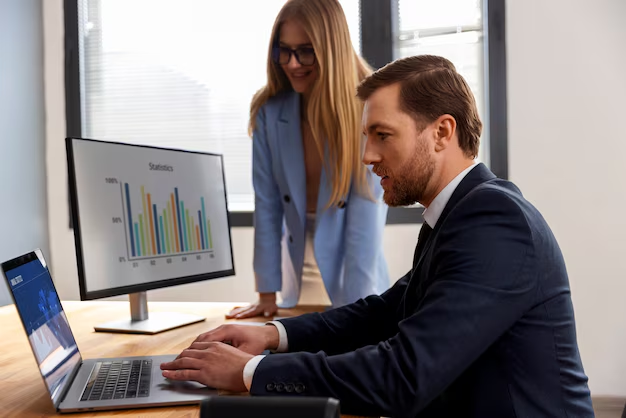
Why Businesses Trust Our Sentiment Analysis
Clients work with Pearl Lemon AI because we understand industry-specific nuances and deliver solutions built for real-world application.
Our systems feature:
- Domain-specific training datasets for better relevance.
- Integration-ready tools compatible with CRMs, BI dashboards, and ERP systems.
- Compliance with GDPR and ISO data security standards.
For instance, we’ve developed systems for retailers that connect directly with their supply chain tools, ensuring sentiment insights lead to actionable changes like inventory updates
Make Data Meaningful With Pearl Lemon AI
Turn unstructured feedback into measurable insights that help you stay ahead of the curve. With Pearl Lemon AI, you gain the tools needed to monitor sentiment shifts, improve customer interactions, and inform strategic decisions.
👉 Request Your Sentiment Demo Today and take the first step in understanding your audience like never before.
FAQS
Our models leverage transformer-based architectures such as BERT, GPT, and LSTMs. These ensure context-aware interpretations of text, delivering high accuracy across varied datasets.
Our tools incorporate custom NLP pipelines designed to process modern digital language, emojis, and abbreviations, achieving over 92% accuracy.
Yes. Even datasets under 1,000 entries benefit from our pre-trained models, which ensure quality insights even with limited data.
Yes. Our systems integrate directly with live data feeds, offering sentiment updates in as little as 2 seconds.
This advanced method focuses on identifying sentiment tied to specific features, such as “price” or “quality” in reviews. It’s especially useful for industries like retail and hospitality.